How did a piece of technology born from niche military interests develop to a point where it could translate medical texts with an impressive accuracy rate of up to 94%?
With the global machine translation market size today estimated to reach 3 billion USD by 2027, it’s a good time to explore what is machine translation. How did we get here? And how does the future and potential of MT shape ours?
I will try to explore all of this and give you the answers you may seek.
Overview
- ~1.1 What are the advantages of machine translation?
- ~1.2 Why do people use machine translation?
- ~1.3 Why is machine translation important
- ~3.1 Types of machine translation
- ~~Rule-based MT
- ~~Example-based MT
- ~~Statistical MT
- ~~Neural MT
- ~3.2 What types of machine translation are there in 2021?
- What are the difficulties of integrating machine translation into your work?
- How can you benefit from using MT in a professional environment?
- ~7.1 Pairing machine translation & human review
- ~7.2 Using a translation management system with integrated MT
- ~7.3 How to use machine translation in Redokun
1. What is Machine Translation?
Machine Translation (MT) involves the use of computers to translate one language (the source) to another language (the target) without human intervention.
These computers are programmed to perform translations using complex algorithms, neural networks, or a mix of both.
The most famous application of MT today is surely Google Translate, but it's not the only product available and, in some cases, it might not be the best.
To understand why MT is projected to be a multi-billion dollar industry in a few years, let's look at its benefits and possible applications.
Want to understand machine translation in a heartbeat? — here's a short 1-min video of how machine translation work in Redokun:
1.1 What are the advantages of Machine Translation?
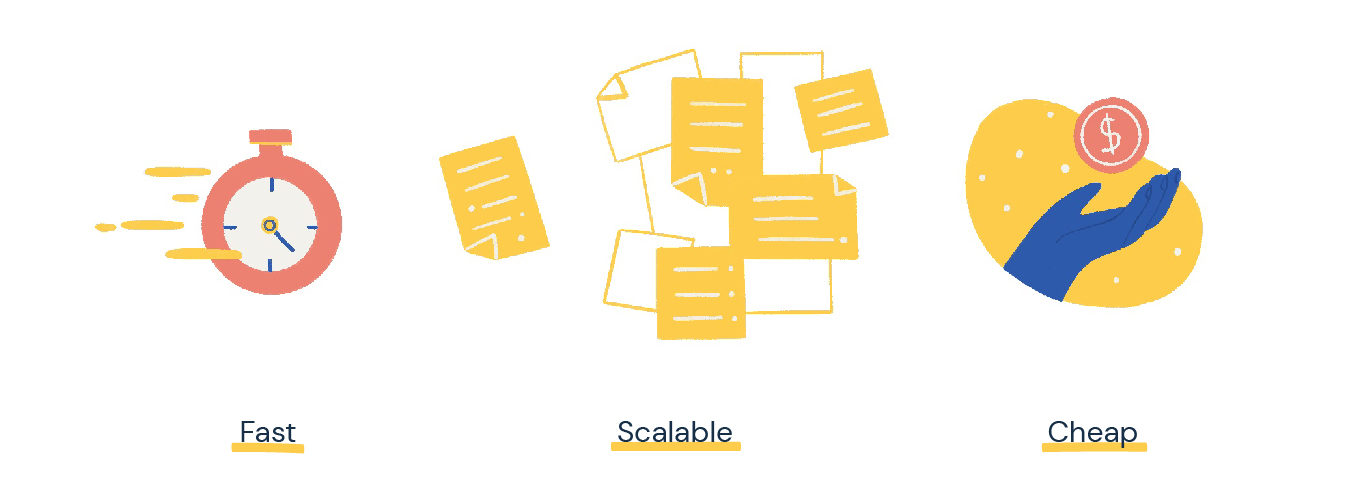
- It's fast: Speed is the name of the game in MT, which translates any kind of content almost instantly. This is the one area where we humans are naturally unable to compete with machines.
- It's scalable: MT engines can return a translation in a matter of seconds whether you're translating a single sentence, a paragraph, or a document. This advantage is especially important to multinational businesses that translate a significant amount of content for internal and external use.
- It's cost-effective: While most of us peasants might have used Google Translate for free, did you know that there is a paid version as well? At the time of writing this piece, the cost to translate 500,000 characters is $10. In comparison, the cost of human translation, which varies between $0.08 to $0.25 per word, is 800 to 2,500 times more expensive than Google Translate premium.
1.2 Why do people use machine translation?
Okay, let's address the elephant in the room. Machine translation may be astonishingly fast but the quality could also be astonishingly bad. So where is it actually used and why?
People generally use machine translation for three reasons: assimilation, communication, and dissemination. And as you'll see below, they don't always need a perfect translation output to achieve these goals - as long as it's fast, scalable, and cost-effective.
1.3 Why is machine translation important?
Overall, people use machine translation to varying extents for different reasons. Since the technology has been expensive to research and develop, why even put money into something that may not achieve perfect accuracy?
Well, even in its current state of "imperfection," machine translation is an affordable tool that serves both commercial and humanitarian interests.
Being able to engage with parts of the world that speaks a different tongue presents new opportunities.
For businesses, it's the chance to reach potential customers and become more productive (more on this later).
For humanitarians, it's the ability to share key information when and where it is most needed, especially in education, healthcare, and relief efforts.
As the nonprofit organization Translators without Borders noted, even “basic phrases can save lives in a humanitarian emergency, yet often communication fails because humanitarian aid workers and the people affected do not speak the same language.”
Whichever side of the coin you land on, business or altruism, you share the goal of helping people in some way. And in order to accomplish this goal efficiently, it's ideal to possess a quick and inexpensive method to translate and convey your most crucial messages.
Machine translation is that method. It may not be perfect now - or ever - but it is still useful to have MT in certain contexts than not.
2. How machine translation became this good?
The short answer would be decades worth of research motivated by different reasons from military advantage to commercial viability.
The long answer you'll find in this timeline of major machine translation advancements from the beginning until today.
Before the Computer
- 9th century, Iraq – Al-Kindi, father of Arab philosophy, developed some techniques for systemic language translation, which laid the groundwork for modern MT. (Source)
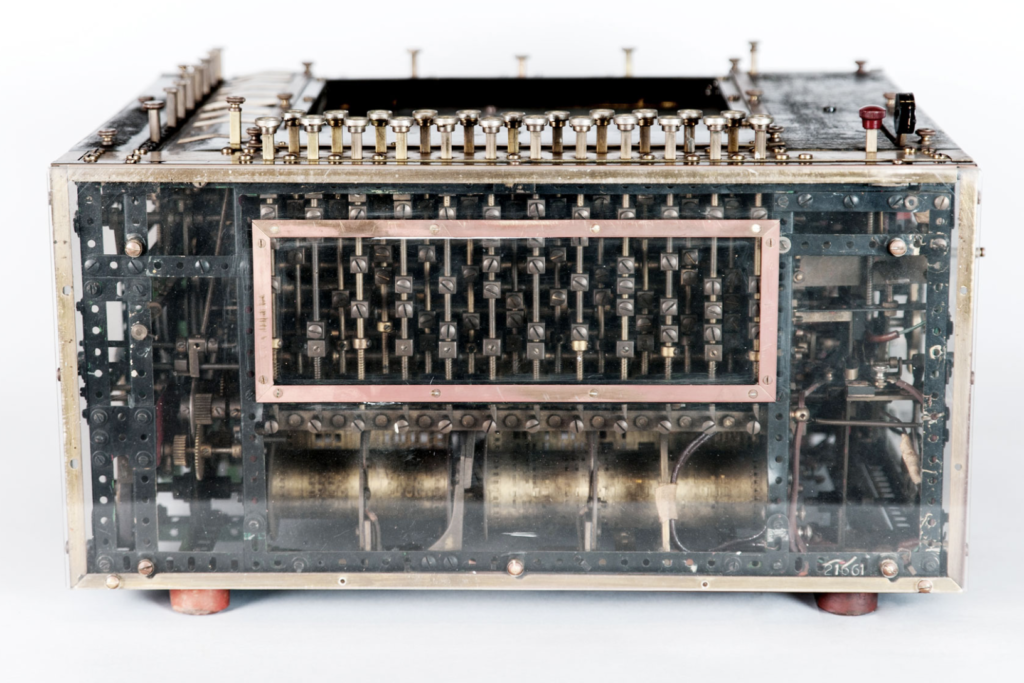
- Early 1930s, Paris – The first patent for a “translation machine” was filed by Georges Artsrouni, a French engineer. His invention was a multipurpose storage device that could retrieve and print stored information. Thus, he claimed that this “mechanical brain” could serve as an automatic bilingual dictionary, among other applications. (Source)
- 1933, Russia – Russian scholar Peter Troyanskii proposed a more detailed device that combined a bilingual dictionary with a method to account for grammatical roles between languages. However, Troyanskii's pursuits to mechanize translations were never completed due to health issues and his work remained largely unknown until the late 1950s. (Source)
From Cold War to Globalization
- 1949, USA - American researcher Warren Weaver wrote the "Translation Memorandum," which is regarded as one of the most influential pieces of literature in early MT development. At a time when computing technology was still in its infancy, Weaver proposed innovative frameworks of using computers to translate documents that notably deviated from the limited word-for-word approach. (Source)
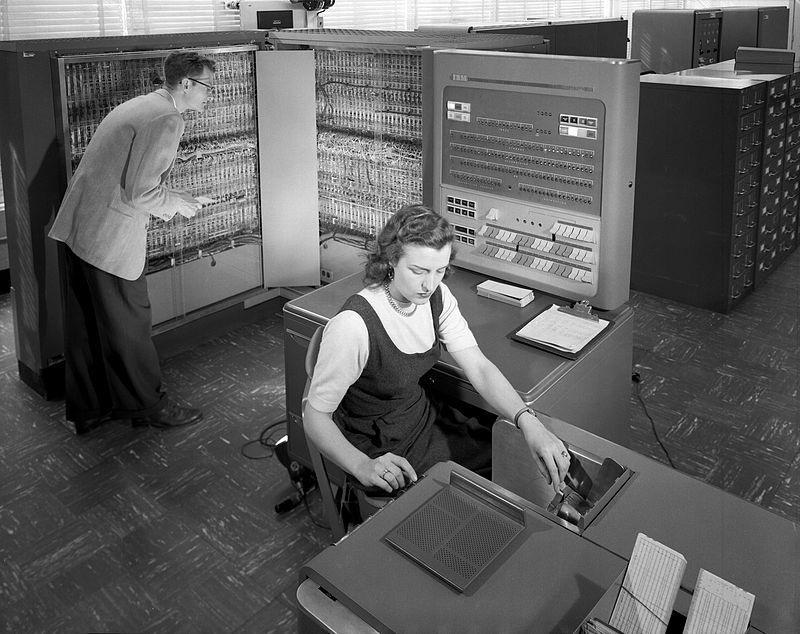
- 1954, USA - Weaver's proposals inspired intense research at universities throughout the country. These efforts culminated in the Georgetown-IBM experiment, a public demonstration of MT where 49 pre-selected Russian sentences were translated into English for the first time in history.
This achievement, which sparked worldwide interest in the potential of MT, prompted Canada, Germany, France, and Japan to join the race to develop the technology. (Source)
- 1960s, USA & Soviet Union - MT research was mostly focused on Russian-English scientific and technical documents. Both countries were pursuing confidential information, which would then be sent to a human translator for a better translation.
Since the technology was mainly developed for military interests, MT in the 60s worked with limited input and language pairs. (Source) - 1966, USA - The ALPAC report was published, expressing great concern over the lack of progress in MT research despite significant investments. MT seemed to have reached a plateau at the "automatic dictionary" stage that was severely limited.
- 1968, USA - With decreased funding, US researchers dropped from the race for MT for almost a decade - one of the exceptions being Dr. Peter Toma who founded Systran.
- 1970s, General - In the 70s, MT research started to lean towards developing low-cost systems that could translate a wider range of documents. This is due to the shifting demands from military to globalization and commercialization.
- 1970, USA - The Systran system was installed by the United States Air Force during the Cold War. Like many other systems of that time, Systran was based in machine translation (RBMT), which drew from bilingual dictionaries and transformation rules (aka grammar).
- 1976, Europe - The Systran system was also installed by the Commission of the European Communities.
- 1977, Canada - The METEO System, developed at the University of Montreal, was installed in Canada to translate weather forecasts from English to French. The system would remain until 2001 and at its peak, it was translating nearly 80,000 words per day or 30 million words per year.
Rise of Microcomputers and Commercialization
- 1980s, General - By this time, machine translation systems have diversified while the increased availability of low-cost microcomputers also paved the way for the development of lower-end MT systems.
- 1984, Japan - Computer scientist Makoto Nagao came up with the idea of translating using analogies and ready-made phrases - known as example-based machine translation (EBMT) today. This approach worked by categorizing, comparing, and combining the phrases within a sentence in both the source and target language.
- 1990, USA - IBM presented a statistical machine translation (SMT) model that has no preconceptions about linguistic rules. Instead, the system analyzes bilingual text corpora and then generates statistical models to predict the best possible translation. At first, the SMT model was word-based but going by words alone often fails to account for context. Phrased-based and syntax-based SMT are more common these days.
- 1994, USA - Corporations (including the long-standing Systran) started showing vested interest in offering machine translation on personal computers and workstations. The advent of the Internet created more demand for translations.
- Late 1990s, General - Machine translation sites became available on the Internet, making them even more accessible for the average user. Notable ones include AltaVista's Babel Fish and Google Language Tools - both of which were exclusively using Systran technology at the beginning.
The 21st Century
- 2007, General - Google switched from Systran to launch its own MT but the translation quality is not that great yet.
- 2016, General - Google Translate started using its own neural machine translation (NMT) system. The system generates translations by creating artificial neural networks that mimic how the human brain learns and stores information.
- 2017 - DeepL Translator started using a new and improved architecture of neural networks. Many agree that the translation results were more natural compared to other MT providers.
- 2020 - Facebook creates the first Many-to-Many multilingual translation model that can translate directly between any pairing among 100 languages without relying on English data.
- 2020 - DeepL reported huge improvements in translation accuracy. The company also added support for Chinese and Japanese translations with unprecedented accuracy. Neural MT seems to be the new state-of-the-art.
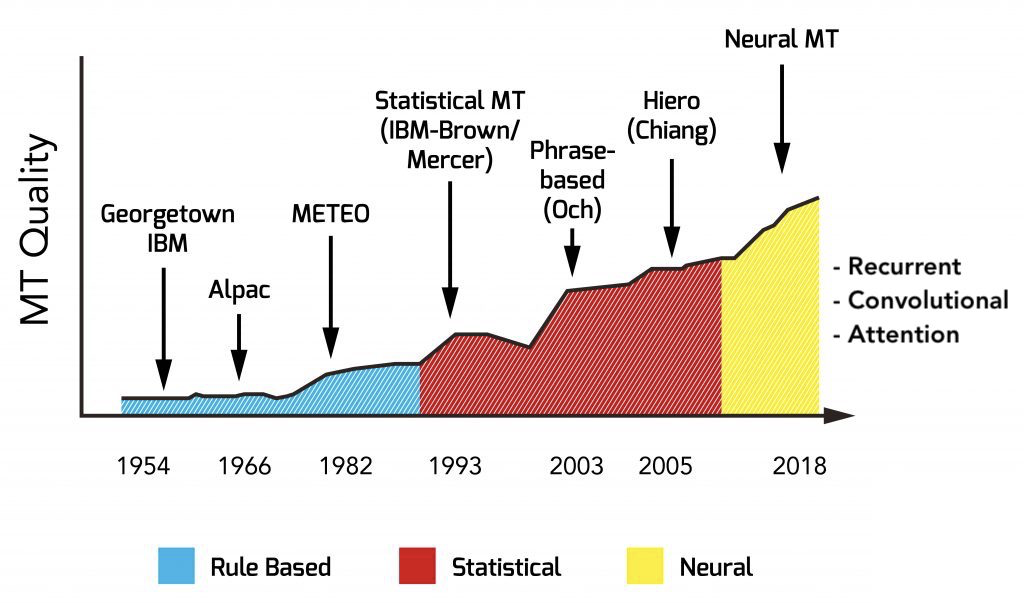
3. How does Machine Translation translate the text?
Machine translation is rooted in systems - and there are different schools of thought that influence how this system should be.
As new perspectives about the structure of natural languages emerge, these systems tend to become increasingly nuanced and complex, and sometimes they are combined.
In the past, we've had MT systems based on sets of rules, analogies, or statistics, which didn't always manage to produce natural-sounding output.
Newer MT frameworks tend to mimic how the human brain operates in recognizing and creating relationships between language pairs.
Let's explore the 4 major types of Machine Translation and how they work.
3.1 Types of Machine Translation
- Rule-based machine translation (1950 - 1980)
- Example-based Machine Translation (1980 - 1990)
- Statistical Machine Translation (1990 - 2015)
- Neural Machine Translation (2015 - Present)
Rule-based machine translation - RBMT (1950 - 1980)
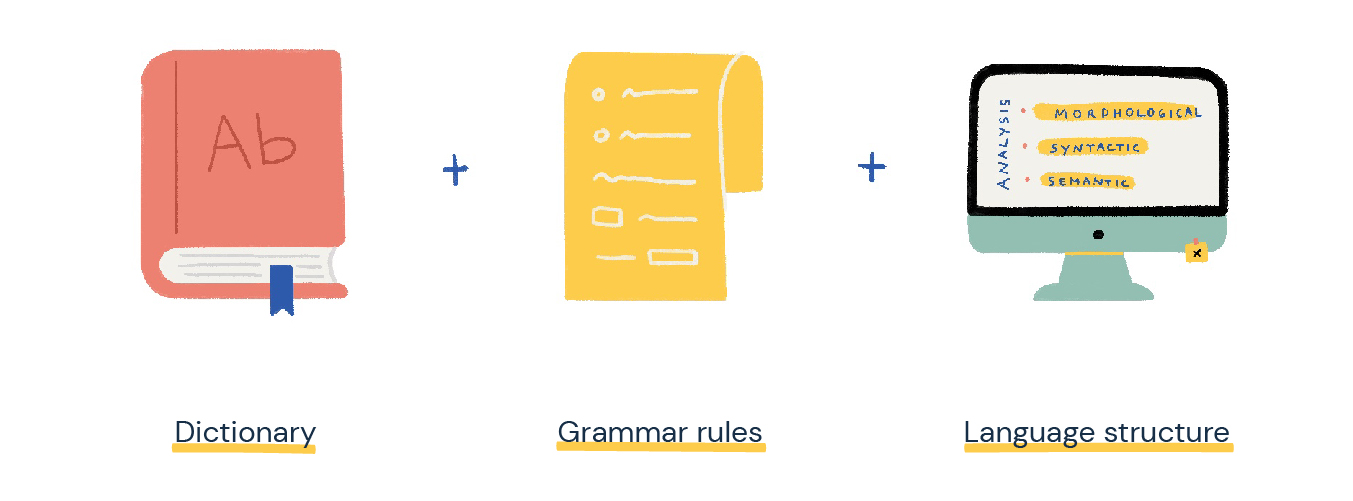
- This is also known as the "classical approach" to MT.
- RBMT systems draw input from dictionaries and grammar rules, which cover the fundamental linguistic features of the source and target languages.
- They then attempt to link the semantic, morphological, and syntactic structures of both languages in a pair to generate a translation.
- Additional rules often have to be added to account for exceptions, names, spelling, and unique characters.
- Examples of RBMT include Systran (1970) and the Météo System (1977) for weather forecasts.
- Issues: Although every error in translation output can be corrected by adding a new rule, these rule interactions can be inconvenient and expensive to implement on a larger scale. There is also a lack of good dictionaries from which to draw data.
Example-based Machine Translation - EBMT (1980 - 1990)
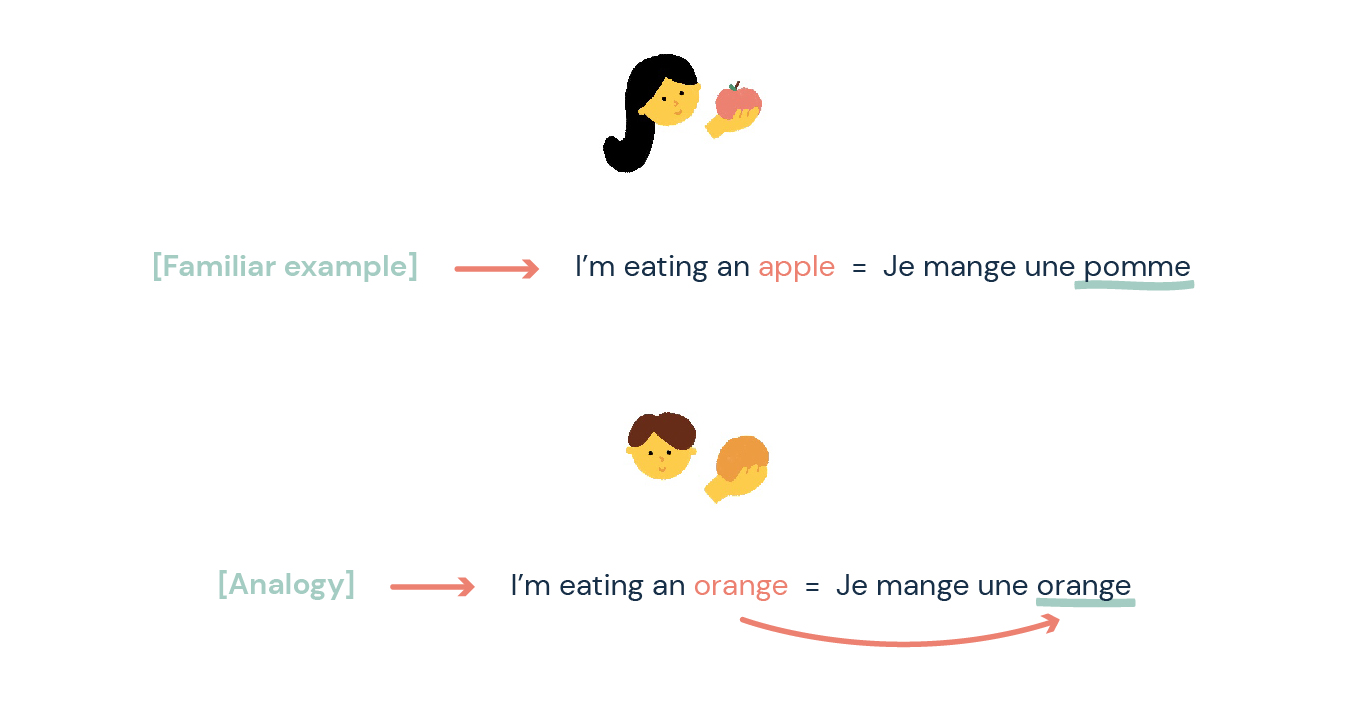
- EBMT is essentially a translation by analogy. The system is provided with examples of correct translations that it uses to solve a new translation.
- As such, this approach requires a bilingual corpus with parallel sentences.
- During translation, the system breaks down a source sentence into smaller phrases and matches them against the examples given. When there is a match, the specific phrase can be substituted with the corresponding target phrases from the example.
- To illustrate, the machine can translate "I am eating an apple" by matching the components against an existing translation of another sentence "I am eating an orange." Once it figures out the similarities, it only needs to translate what is different.
- An example of EBMT is Translation Memory, which is still widely used commercially today.
- Issues: With little regard for actual syntactic and semantic rules, the EBMT approach relied heavily on using huge databases of references to translate effectively.
Statistical Machine Translation - SMT (1990 - 2015)
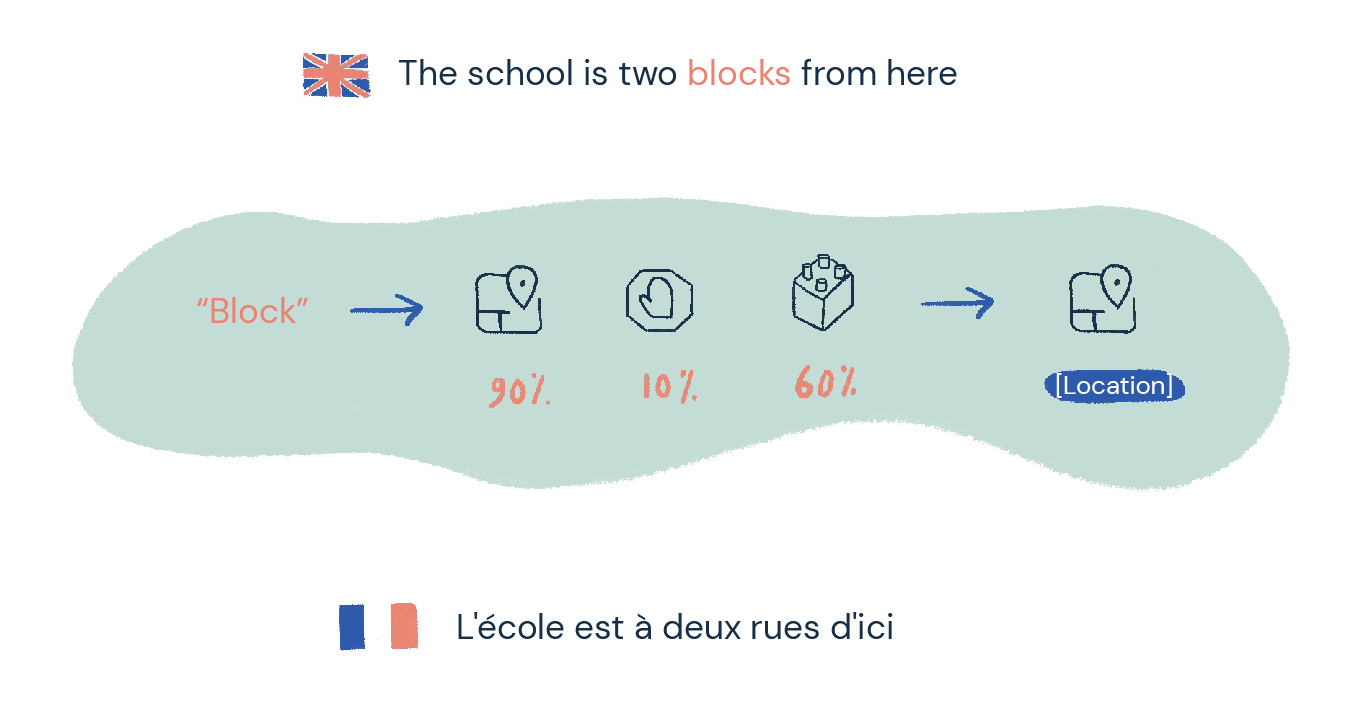
- SMT analyzes bilingual corpora and defining statistical rules from them to produce a translation. Unlike the example-based approach, SMT doesn't just use the corpora as frames of reference.
- Compared to the previous models, SMT can create more fluent translations with a significantly lower cost of development.
- There are three main groups of statistical machine translation: word-based, phrase-based, and sentence-based. The difference is mainly what the system views as the fundamental unit of translation.
- Word-based SMT is limited when it comes to homonyms and words with no equivalents in the target language. Phrase-based SMT aimed to reduce these limitations by recognizing blocks of words as a unit.
- Finally, syntax-based SMT utilizes syntactic units, which was better at translating between languages with distinct syntax structures. Once considered “the future or translation,” this approach has largely been overtaken by new technologies (Neural Networks and Deep Learning).
- The most famous SMT is Google Translate (before its 2016 update) which was sentence-based.
- Issues: SMT systems are less capable of handling cases, gender, ambiguity, and idiosyncratic expressions.
Neural Machine Translation - NMT (2015 - Present)
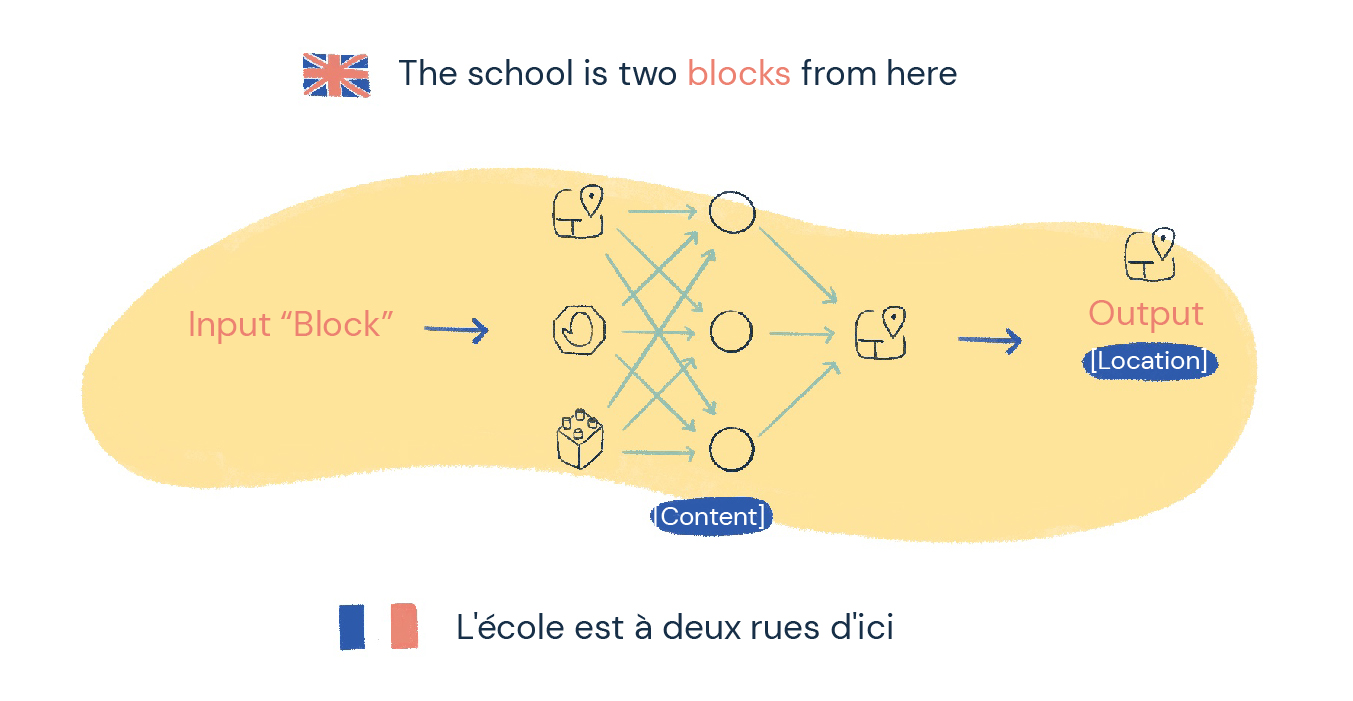
- Based on artificial neural networks, NMT presented an approach that's capable of harmonizing gender and case between different languages.
- NMT basically imitates the behavior of our brain in connecting and encoding information to learn mathematical functions for translation.
- Neural systems typically use an encoder-decoder structure whereby textual data is first encoded into numerical representations, which are then passed to a decoder to generate a translation output.
- Unlike in statistical MT, a neural MT system does not consist of small sub-components that have to be calibrated separately. Instead, it establishes one vast network of interconnected components, which the system continues to fine-tune as it is continuously used.
- Today, neural networks and deep learning are the driving force behind state-of-the-art MT algorithms.
3.2 What types of machine translation are there in 2021?
Neural networks have become the norm in machine translation in 2021 - with leading platforms like Google and Microsoft embracing the technology,
However, any type of MT system will have its strengths and flaws. For example, in neural MT, short sentences are still somewhat difficult to get right while statistical MT tends to achieve higher accuracy in this regard.
To improve machine translation quality further, the industry has adopted some new methods, such as:
- Hybrid machine translation, which often involves combining NMT and SMT for better output;
- Adaptive neural machine translation, which adds layers of context and a real-time feedback loop to standard NMT systems. This is particularly useful for business use because the MT would be able to capture the unique voice, tone, and style of a brand based on translator or reviewer feedback.
4. Why Machine Translation is difficult?
Here are a few reasons why MT algorithms are so difficult to perfect.
1. Words and sentences can be ambiguous. When there is more than one possible meaning, we can try to solve the issue by using statistics but it isn't always precise. Disambiguation is a strategy whereby the AI attempts to make sense of an ambiguous word by analyzing the context through statistics to different extents - and with varying degrees of accuracy. The disambiguation process comes naturally to us humans whenever we're reading or listening. Without us realizing it, our brains easily activate deep layers of context and intertextuality when we encounter ambiguous language. Training MT systems to work in a similar manner will certainly be an expensive endeavor.
2. Translation sometimes requires a deeper analysis of discourse structures. Consider the meaning of "since" in the following two sentences:

In the first sentence, "since you brought it up" specifies a condition for the second half of the sentence, whereas in the second sentence, it serves as a measure of time. Producing correct translations for either depends on whether the MT is able to analyze the context and distinguish the sense of the word "since."
3. There is insufficient data for non-standard language compared to standard language. MT systems have to be trained by feeding them linguistic information but there's often not enough data for colloquial language that's generally not used in publications. As a result, we can train the MT to translate a text with high levels of accuracy but only if the source text is grammatically sound. However, that wouldn't be a realistic representation of all types of content that needs to be translated, especially casual speech and creative copies.
4. Similarly, there is also a lack of data for certain languages that are not well-documented, making it difficult to train the MT.
5. Named entities, as well as expressions of time, space, and quantity, can be difficult for the MT to handle. Manual corrections to the MT system or the output may still be required when it comes to distinguishing proper nouns from common nouns.
6. Word order may change drastically. Since languages have different syntax structures, the process of aligning them may cause issues particularly when there are complicated sentences. A basic example would be the English-Japanese translation pair. English follows the subject-verb-object order whereas Japanese is subject-object-verb language.
7. Translating pronouns require different strategies. One of the ways to handle pronouns is shown here:
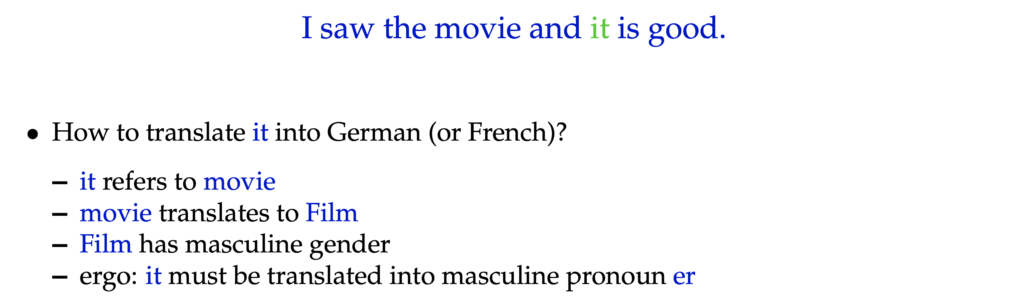
Of course, this isn't the only method. Human translators may also employ other strategies like omitting the pronoun and changing the pronoun to its reference. The challenge now is to train the MT to know when to use each method.
8. Coreference is hard. This occurs when there are two different terms that point to the same person or thing, such as "his daughters" and "cousin" in the following example.

Problems may arise when the MT tries to translate "cousin" correctly for certain target languages like Italian, where "cousin" can either be translated to the female variation "cugina" or the male variation "cugino". We must somehow train the MT to deduce that the correct translation for the example above is "cugina" due to its coreference.
9. A sentence can be translated in multiple ways and sometimes the right choice could be subjective. Even if MT can achieve a high accuracy rate grammatically, perceptions of the translation quality could still differ from person to person. This could happen in cases involving synonyms, such as:
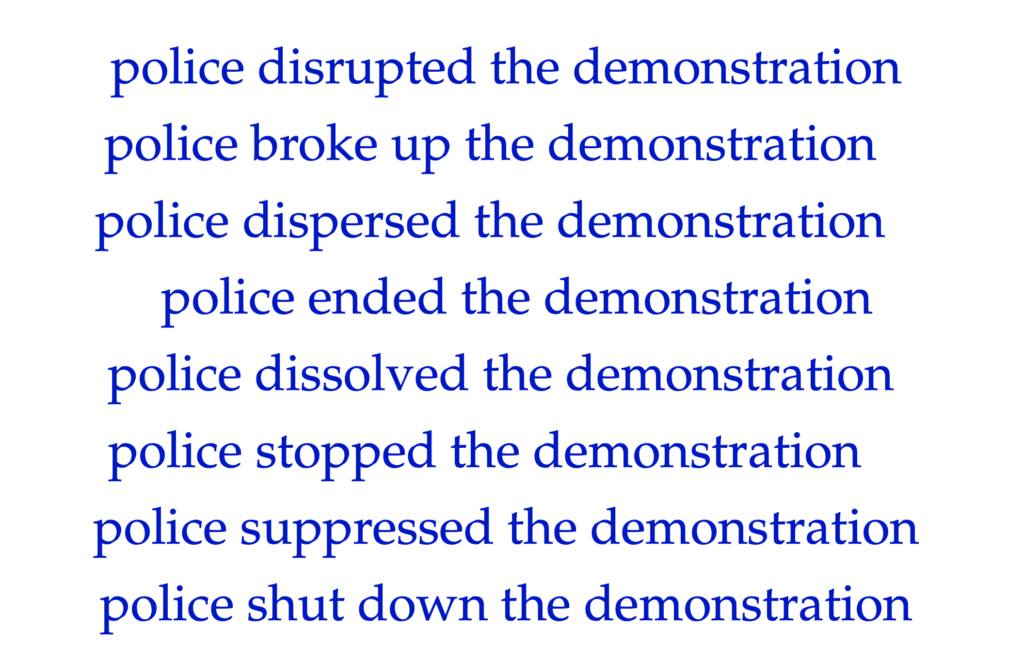
And in other cases, it could simply be a matter of style, which means there is no one right answer as shown here:
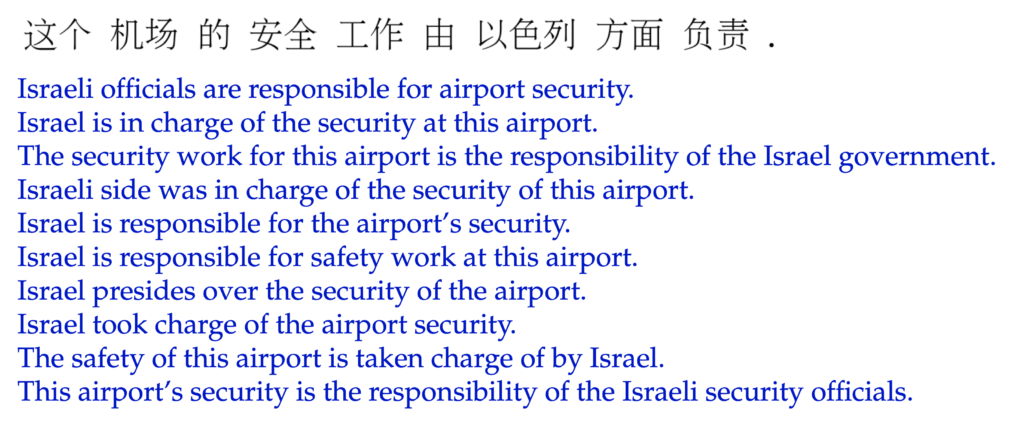
5. How good is machine translation today?
Good question! Considering the complexities of translation we just mentioned, the answer is...it depends on the language pair and the context of use.
A 2021 study found that Google MT was able to translate medical instructions for patients with an accuracy rate of 94% for Spanish, 90% for Tagalog, and 82.5% for Korean.
While these numbers look promising, MT is still not good enough on its own in the clinical setting because errors in translation have higher stakes. They could potentially harm a patient.
In another study involving English to Swedish translation of an opinion piece, the MT output required an average of 3 edits per sentence. It was also reported that the human translation of the same text was generally longer and structurally varied.
What you might get from these numbers is that a lot depends on the language pairs you are dealing with, as well as the type of content you need to be translated.
Machine translation isn't comparable to human translation but it has many practical applications in the right context. That's one reason why the MT industry is growing so much and so rapidly these days.
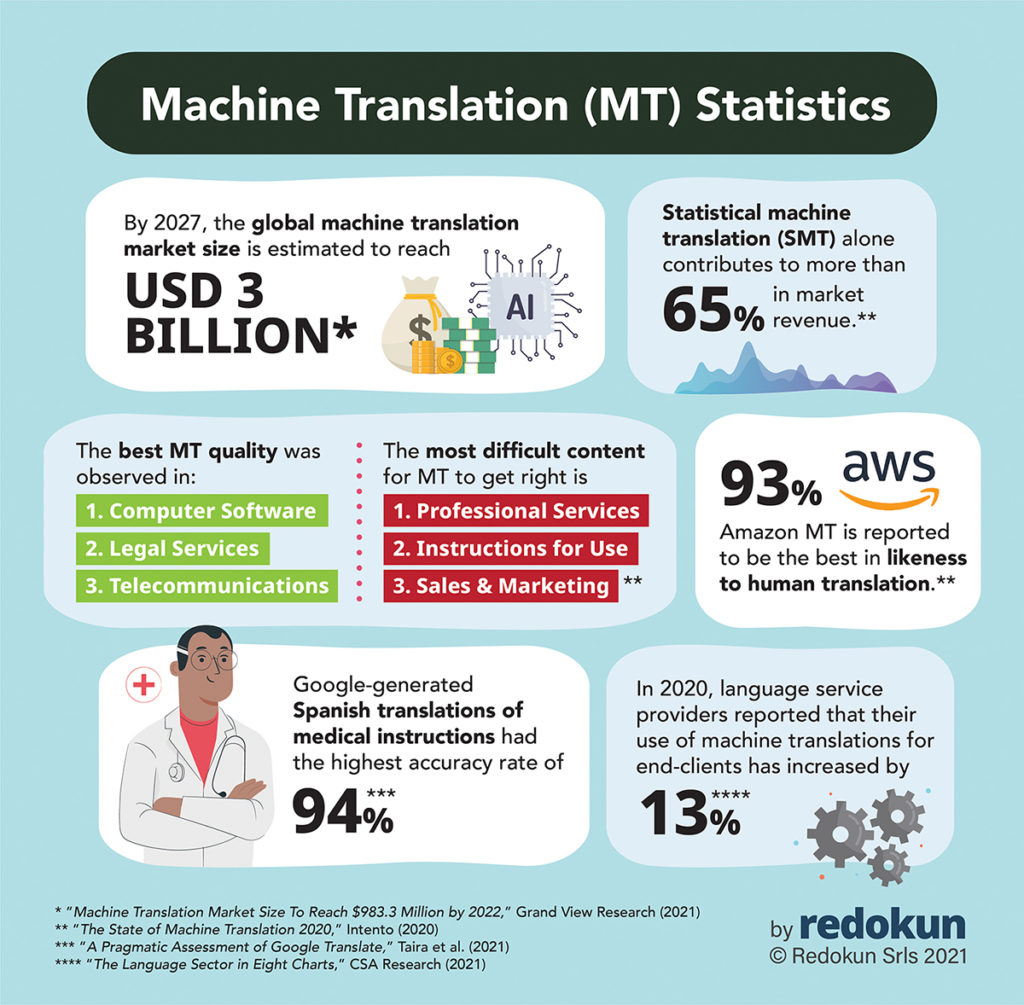
5.1 Do companies use machine translation?
Machine translation has many uses in business and professional settings. With globalization in full swing, many companies use MT to enhance their systems and fulfill their internal and external communication needs.
For example, multinational budget airline company AirAsia uses MT to facilitate communication among their 22,000 employees from 16 different nationalities - many of whom do not speak English.
MT is also instrumental in helping businesses translate large amounts of content for their target audience overseas without excessive effort and localization costs.
5.2 What is the best machine translation?
No single machine translation engine is the best at everything. The output quality of the MT you choose will depend on what you are translating.
In another post, we analyzed some of the best machine translation software for enterprise. Here are the MT providers we prefer and integrate with at Redokun and why:
- Google Translate: They support more languages than any other service provider, and the translation quality is generally high.
- DeepL: Although they only cover 13 languages, they are usually the best in the industry at translating those 13 languages.
Machine translation technology for businesses is usually built into other types of software products (like Redokun). As such, the best MT for you or your company is also a question of what you want to achieve with the tool.
For instance, if your goal is to improve the accessibility of information related to your product or service for worldwide audiences, you could look into help desk solutions that support MT.
On the other hand, if you want to speed up how your team translates sales material, collaterals, digital media, and other assets, you'll want to look into a tool that either already supports or can be integrated with MT.
Here are some helpful guides about translating Word documents, Excel sheets, and PowerPoint presentations.
6. What are the difficulties of integrating Machine Translation into your work?
Depending on your experience with localization solutions, there could be a few hurdles to overcome before your team can start reaping the full benefits of MT.
6.1 Setting up your MT solution
The first steps toward implementing a machine translation engine can be a pretty difficult task for the casual user. You would need to create an account with the MT provider, set up their APIs for your systems, then deal with subscription and payment.
If you're unfamiliar with the process or simply wish to skip it altogether, the alternative is to find a software solution that enables you to use the MT engine of your choice without additional setup.
A good example of specialized software with built-in MT is translation management systems (like Redokun). They essentially help you optimize your entire localization workflow with machine translation as an added feature.
6.2 Evaluating your options for MT
Off the top of my head, I can think of 15 different machine translation providers, and some companies may not have the time or expertise to evaluate all of them.
While we've specifically written a detailed guide on how to choose a translation management system, the same principles still apply to selecting the right MT service for your business.
Start by answering these simple questions that probe into what your current workflow needs:
- What is your main reason for using machine translation? Will you mainly use MT to understand foreign documents or communicate with your customers through live chat? Or do you want to translate professional websites and publications where a higher translation quality is necessary?
- What type of content do you need to translate? Documents, applications, help desk, or something else? Your answer will determine the type of MT-enabled software you need.
- What language pairs do you need to support? You can further narrow down your options based on which MT provider performs the best on certain language pairs.
6.3 Understanding how MT fits into your workflow
Machine translations often get a bad rep because people tend to compare them to translations produced by real people, creating unrealistic expectations in the process.
In its current state, MT technology isn't meant to replace your team but to make their jobs easier. In the areas where MT does shine, namely speed and cost, there are useful applications for businesses looking to become more productive at translating tons of content for global consumers.
When dealing with a particularly large or complex piece of content, MT can help your team run that first leg of the race by translating the entire thing in a matter of seconds. So all that's left to do is to fine-tune the output.
In other words, MT software sets a good pace for any project because you have something substantial to work with as opposed to starting from scratch.
6.4 Managing Data Confidentiality
MT tools will need access to your data in order to generate translations. Some MT providers may even use your data to train and improve their engines.
At Redokun, confidentiality is something we're extremely mindful of because we're handling third-party data.
When you upload your content to Redokun, we make sure that our MT providers only keep your text temporarily for the sole purpose of translating it. It will be deleted from their database once translations have concluded.
This is just one of the key measures we take to protect your data and other providers may do the same. The bottom line is to check the terms of service before subscribing to any software, especially if you work with sensitive and confidential materials.
7. How can you benefit from using MT in a professional environment?
7.1 Pairing Machine Translation and Human Review
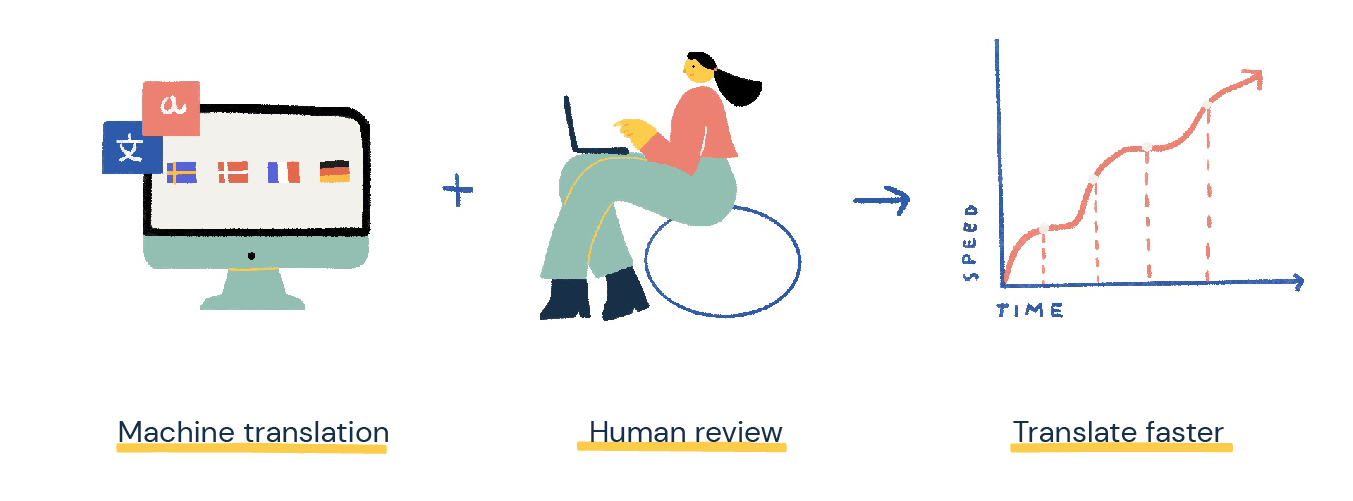
You can benefit from machine translations by using them as a reference rather than a replacement for actual translators.
Natural languages are dynamic and creative - even the best human translators will struggle to capture and transfer their meanings from one language to another quickly.
This is where many localization teams often start to lose momentum and productivity; more so when a project involves a large amount of text.
In these situations, machine translation offers your team:
- Instant translations that can be used without revision for simple sentences;
- Immediate insight into key semantic and grammatical components of long or complex sentences;
- Base structures to build a more coherent translation from.
In other words, your team will essentially be doing more post-editing than translating intensively. When implemented correctly, this process could mean reduced translation costs and a faster time to market.
Businesses make the most out of MT when it goes hand in hand with human review, which serves to overcome the "blind spots" of MT, such as ambiguity and unique use cases.
7.2 Using a translation management system with integrated MT
As mentioned earlier, one quick and easy way to set up machine translation services for your team is to get a translation management system that already includes MT.
Many of our clients here at Redokun use our integrated MT technology to empower their daily localization tasks. If you're planning to set up something similar for yourself, here are the MT tools they're using on Redokun and how they use them:
- Translation Memory: This is an example-based machine translation that keeps a unique bilingual database of every content your team has ever translated, then uses that information to suggest translations for new work.
- Neural Machine Translation: We offer Google and DeepL integrations at Redokun. This type of machine translation uses deep learning to generate translation suggestions.
7.3 How to use Machine Translation in Redokun
Our clients have two different methods of utilizing the tools mentioned above.
The first method is to...
Have a fully automated pre-translation stage. Whenever the client uploads a new translation project, they have the option to pre-translate the entire text using their translation memories (for repeated content) and then our neural MT engines (for completely new content).
After that, the client invites a translator or anyone they see fit to review the draft generated by the MT. The overall process would go like this:
Translation Memory → Machine Translations → Human review → Translated document
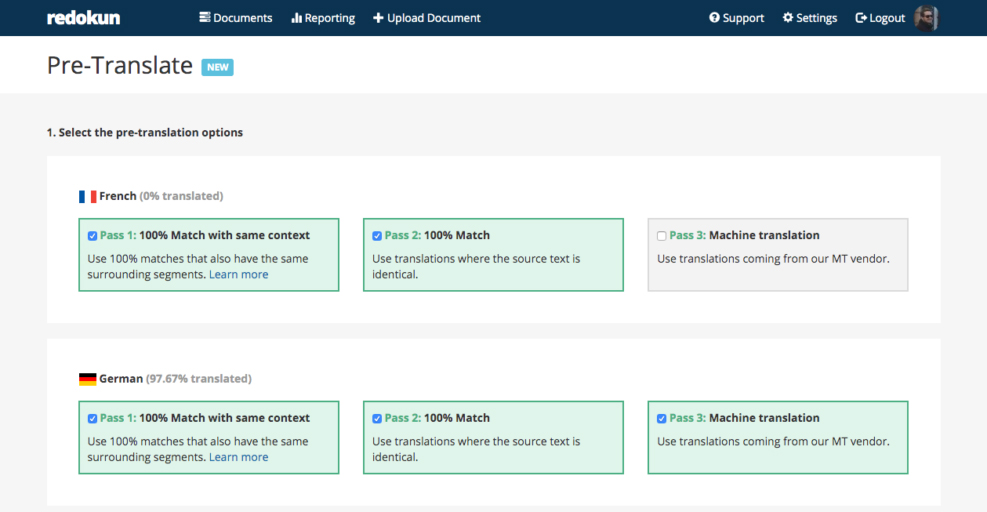
The alternative method is to...
go straight to the translation editor interface and generate MT suggestions as they reach each segment that needs to be translated.
Whenever they upload a new piece of content, Redokun divides the textual information into smaller segments for easy viewing and navigation.
The suggestions from our MT engines appear under the translation box for each segment, which the translator can simply click on to use or revise.
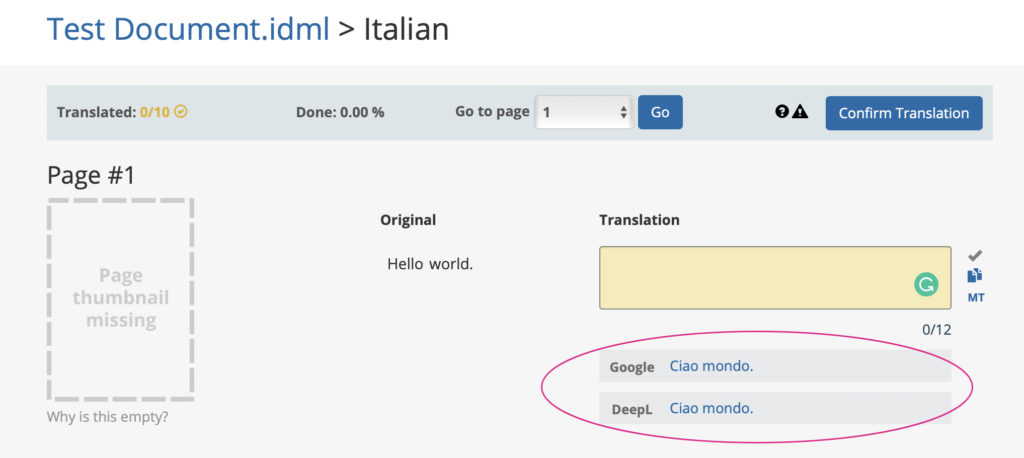
That being said, if you'd like to try these features with your team, here's an invitation to register for a 14-day free trial of Redokun today.
Redokun: Have a Sneak Peek
Want to see Redokun in action? Have a sneak peek at how Redokun works without any commitment:
Conclusion
Although we're not at the stage where we can expect machines to take over the translation process completely, MT technology has already achieved plenty since their output can normally be used after a round of reviews.
Even translations made by humans have to be reviewed at the end of the day. Adopting MT tools doesn't mean we're diminishing the importance of involving real people in creating great content for real people. They simply add an element of speed that was noticeably missing before.
As we continue to try and push the boundaries of what MT can do, let's also remember how the technology is already making our lives much easier today in both work and play.
Till next time,
Shu Ni